Welcome to the Enable Medicine Blog!


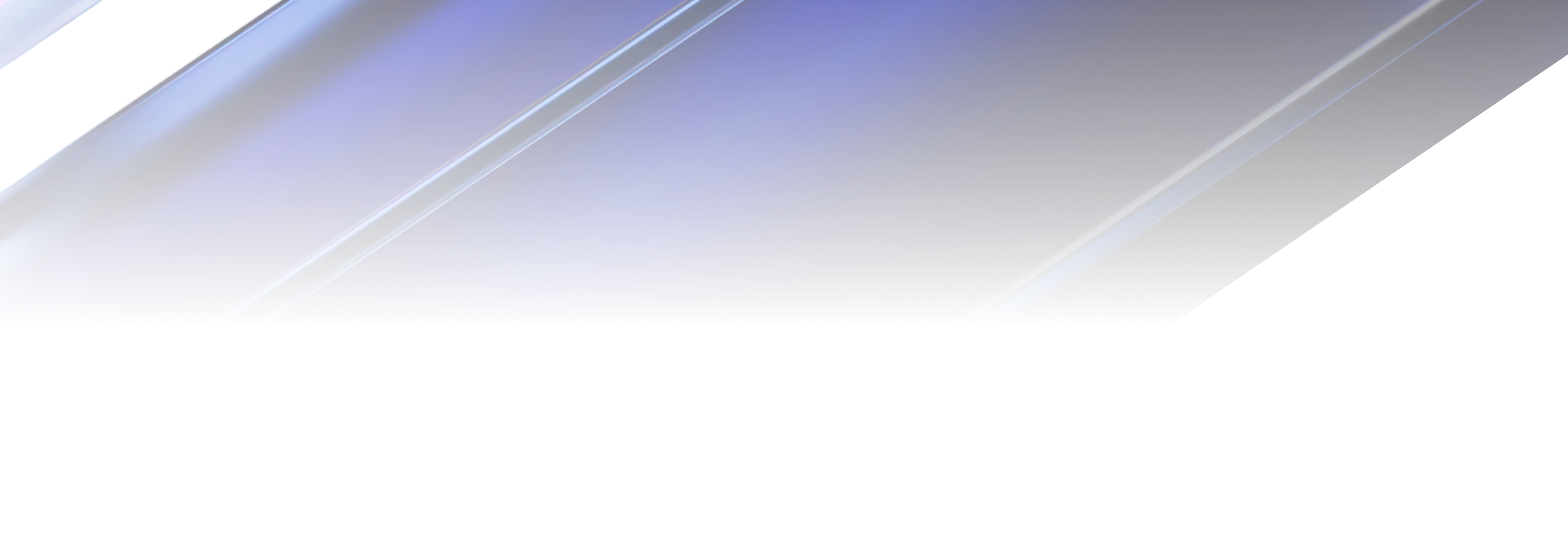
I’m so excited to kick this blog off! I’m relatively new to Enable Medicine(I joined about two months ago), and this place is wonderfully energizing. My colleagues here are so impressive and kind, and the atmosphere at Enable Medicine reminds me a little of my first year of college, where it feels like big dreams and possibilities are just within reach.
The big dream that we’re working towards is to make all of the biological data that has been collected (and will be collected) widely accessible and usable. As with most grand declarations of purpose though, it requires more precision — what exactly does accessible and usable data mean, and what does it entail?
For a clinician, it might mean easily finding treatments and clinical outcomes for patients similar to the patient that you are seeing. For a pharmaceutical company, it might mean looking at histological data to determine why a treatment worked or failed, and using that information to direct further work. For a researcher in fundamental biology, this might mean figuring out whether a particular experiment or observation has been performed previously, to prevent duplicating work and to build upon the results in a meaningful way. For a bioinformatician or an epidemiologist, it might mean interrogating large datasets to discover relevant trends.
Central to all these use cases is the ability to search for relevant information and obtain it in a usable form. While Google has done the former for websites on the Internet, there is not really an equivalent in the realm of biological data. Even when biological datasets are publicly available, they are usually siloed in different publications and access points, making it hard to form meaningful queries against them.
Furthermore, to make the datasets usable, we need to be able to aggregate them in a form that can be easily analyzed and interrogated. This brings to mind data modularity and standardization — both make it easier to combine datasets for higher power analysis, and both require curation to achieve.
Curation is something that I’ve been particularly interested in, since it seems like we’re reaching (or have already reached) a time where we’re inundated with information from myriad sources. The difficulty lies in making sense of all this information, in sorting out the real signal from the noise.
And this is only going to become a bigger and bigger problem in biology: developments in data storage have enabled acquisition and accumulation of increasingly large datasets, and generating large biological datasets is becoming cheaper and easier than ever. As an example, consider the human genome. The first whole human genome (3 billion base pairs, or a minimum of 700 MB and more realistically, 200 GB of data) was sequenced for about $1B USD, but today, it is possible to perform whole genome sequencing for less than $1,000 USD. And that’s only one type of dataset — now, there are large datasets being generated in many various subfields of biology (the -omics fields): transcriptomics, proteomics, lipidomics, histomics, and microbiomics, just to name a few. What can we, as the scientists who generate the data, do to help?
To some extent, scientists already perform data curation when choosing to publish a study and deciding on the presentation of data and the analysis. Many journals also have their own requirements for the presentation and availability of the underlying raw data. However, these standards are often too relaxed to allow for meaningful aggregation of the data, erring in favor of ease of use for the uploader over data availability for the reader (indeed, the state of scientific data sharing merits a whole post on its own). Thus, it remains difficult to search for answers to questions (for example, what is the average makeup of the tumor microenvironment for HER2+ breast cancer patients) across all published datasets and studies.
This isn’t to say that the raw data requirements for publication are useless — indeed, to build this biology platform, having access to raw data is absolutely necessary. It just isn’t sufficient. There needs to be some sort of meta-curation that both aggregates and formats data to be searchable.
Furthermore, from the raw data, it would be nice to have an easy way to apply standard analyses and transformations, both to verify that I can arrive at the same conclusions as the authors and also to explore the analysis space (for example, by adjusting the parameters as appropriate).
All this to say that for a truly universal platform for biology — one that is accessible and usable (i.e., searchable) — we want something that 1) curates the datasets such that they are in a standard format and thus are guaranteed to be in a parsable and usable form; 2) allows scientists to download the standardized datasets to perform their own analyses; and 3) provides standardized analysis tools that easily and transparently run on datasets that can be uploaded to the platform, allowing researchers to adjust the analyses as necessary.
There is one final piece that I want to bring up: a major function of scientific publications is to allow scientists to walk others through their work and place it in context with other studies; likewise, a universal platform for biology should allow the same level of curation. It is this curation from the publishing scientists that enables effective communication between scientists and to the general public.
We hope that by creating this platform for ingesting, operating on, and presenting biological datasets, information can be provided to researchers curated on their own terms and needs. This in turn will increase transparency, decrease costs and inefficiencies, and enable faster discovery and innovation.
In the following posts, I’ll be highlighting some of the aspects of the platform that we’ve developed or are in the process of developing that I think are exciting and interesting, but I’ll have to end here with a bit of a cliffhanger — I hope that you’ll be back for more!

